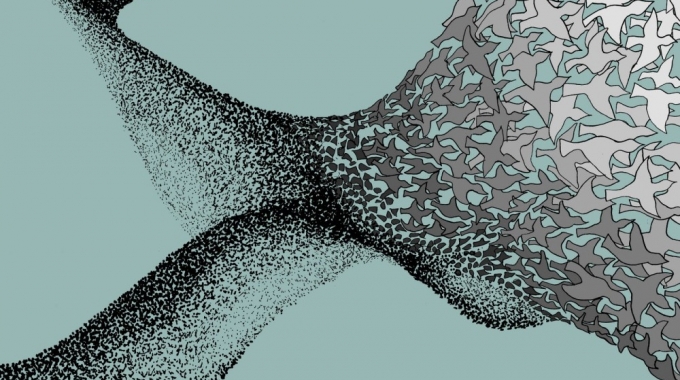
Everywhere you look, animals and people collectively accomplish complex goals that none could achieve individually. Ant colonies efficiently find near-optimal paths to food sources, while anonymous people collaboratively produce sites like Wikipedia. Such phenomena, known as Collective Intelligence, represent an intriguing new paradigm for teamwork and problem-solving. Because these groups are decentralized, they are more creative, resilient, and adaptive than traditional groups that rely on centralized control to make decisions.
I study collective intelligence across multiple domains — from termites constructing mounds to people solving problems over the Internet. Yet while these topics are unified under the domain of collective intelligence, we have no unified way to study them. Instead, scientists studying collective intelligence often operate on a “know it when we see it” principle. This leads to vaguely-defined notions of collective intelligence and makes it difficult to compare instances of collective intelligence.
I have recently been thinking of ways we can define, identify, and measure collective intelligence in a domain-independent manner. Within my research, I’ve found the two traits to be the most fundamental for a group to achieve collective intelligence:
1) The collective outperforms individuals on the given task. That is, groups with multiple agents achieve better results than each could alone. In particular, the ability of the group is higher than the ability of its most able constituent.
2) The collective is more than just a wise crowd. Collective intelligence is often referred to as the “wisdom of crowds” to emphasize the abilities of groups over any individual. But while the two terms are often used interchangeably and there exists overlap between their definitions, we should not conflate the two. Wisdom of crowds occurs when agents solve a problem in parallel and their answers are then aggregated, whereas collective intelligence requires agents to coordinate with one other (or the environment) while working toward a solution.
With these points in mind, I define collective intelligence as follows: Collective intelligence is the ability of a group of agents to improve its ability on a given task by sharing information and responding to cues in the environment while working.
This definition is quantifiable based on the performance of collectives and crowds. The performance of a collective is defined as the quality of its solution relative to the optimal solution. This term is known as the performance above optimal (PAO). Similarly, a collective’s performance relative to a crowd is defined by its performance below a crowd (PBC). These two criteria lay the framework for a Collective Intelligence Index (CII) that could function like an IQ allrec test to compare instances of collective intelligence: CII = (PAO · PBC)−1. Larger values of CII indicate that that a collective displays more intelligence.
As a simple example of how these measures can be applied, I developed a simulation of ant colony optimization (ACO) to solve the traveling salesman problem (TSP). The ACO algorithm was inspired by the foraging behavior of ant colonies, so provides a useful simulation for testing collective intelligence.
Simulated swarms were tested on three sample problems of varying difficulty. Based on my tests, the CII of swarms solving the easy problem is low and does not change for larger groups. The highest CII is observed for swarms solving the medium-sized problem. It increases quickly, indicating the benefits of collaboration, and then remains steady. The CII for the hardest problem is between that of the easy and medium problems. It improves rapidly as swarms increase from 1 to 5 agents, and then steadily grows until it matches the CII of the medium problem with swarms of 25.
My results indicate that the intelligence of collectives depends on the difficulty of their task. The CII increases with group size more for the medium and hard tasks than on the easy task, likely because groups are better able to benefit from coordinating their behavior in more complex environments.
Another interesting result is that although large groups outperform small groups across all tasks, the rate of improvement decreases as group size increases. While groups of 10 significantly outperform an individual agent, groups of 20 perform only slightly better than the groups of 10. Based on these results, one promising route for future research is to determine experimentally how collective intelligence changes for different types of groups.
The notions described here are simple — perhaps too simple to provide a fully unified framework for evaluating collective intelligence.
But for collective intelligence to grow as a science, it is important to continue developing tests and metrics to analyze collective intelligence. Doing so will only yield new insights regarding the nature of collective intelligence and will allow us to further pinpoint specific traits that contribute to collective intelligence.
Want to try being part of a Collective Intelligence? Sign up for the UNU Beta here.
Ben Green is a PhD candidate at the Harvard studying Applied Mathematics and an Academic Advisor to Unanimous AI. You can follow his work here.
Pingback: Be a part of a "human swarm" - Join the UNU Beta! - UNANIMOUS A.I.UNANIMOUS A.I.
Pingback: Collective Intelligence | Pearltrees