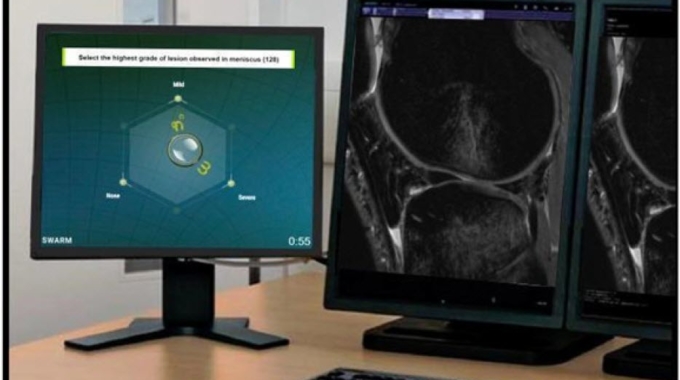
In the field of diagnostic radiology, the interpretation of complex medical images such
as MRIs can be challenging. There is often a high degree of difference in opinions
among experts, even among those with the same level of expertise and experience.
For example, high inter-reader disagreement can be seen amongst experienced
radiologists reading knee MRIs to detect lesions in the meniscus. This leads to an
obvious concern: how can medical professionals give accurate diagnoses if they can’t
agree amongst themselves? This pervasive problem of subjective diagnostic opinion
cuts across Medicine, from mammogram readings in radiology to biopsy diagnosis in
pathology.
A new paper written by Rutwik Shah M.D., and colleagues at the
Center for Intelligent Imaging at University of California, San Francisco (UCSF),
examined this exact problem head-on. They reported significant improvements in
diagnostic accuracy among teams of radiologists using Swarm AI® technology.
Unlike other forms of AI that aim to replace human practitioners with automated
algorithms, Swarm AI is a unique technique for amplifying the collective accuracy of
human groups. Modeled on the biological principle of Swarm Intelligence, Swarm AI
technology enables networked groups to work together to reach decisions, each
connecting from their own workstation while intelligent algorithms monitor their
real-time interactions to determine their relative levels of confidence and conviction.
This creates a closed-loop system where small groups rapidly deliberate and
converge on solutions together.
Specifically, the new paper from UCSF School of Medicine uses the Swarm® software
platform from Unanimous AI. As shown in Figure 1, the platform enables small groups
to answer questions by collaboratively manipulating a graphical puck to select from
among a set of options. Each participant provides input by moving a graphical
magnet with a mouse, touchpad, or touchscreen. By positioning their magnet with
respect to the puck, participants apply their will on the system. The input from each
user is not a discrete vote, but a stream of vectors that varies freely over time.
Because all members adjust their intent continuously, the group reaches a solution,
not based on the input of any single individual, but based on the dynamics of the
system as moderated by the swarming algorithms.
The overall quality of the diagnoses was up to 32% better when using Swarm as
compared to the Plurality vote, Most Confident vote, and the AI model. Moreover,
the number of correctly identified normal cases (true negative) improved
significantly, as opposed to individual readers who were all biased towards
over-diagnosing lesions.
For more information, read the full case study here.